Advanced techniques for counterparty risk modeling can revolutionize your risk management strategies. At the core, ensure your model analyzes financial statements, reviews past credit history, evaluates industry trends, and adheres to regulatory requirements. This multi-faceted approach provides a comprehensive understanding of a counterparty's creditworthiness and potential exposure, shedding light on hidden patterns through predictive analytics.
Machine learning algorithms enhance counterparty risk assessment by analyzing large datasets to reveal subtle correlations and predict defaults more accurately. Feature engineering also plays a pivotal role, transforming raw data into meaningful inputs for your models by identifying relevant variables. Real-time exposure monitoring further mitigates risk by enabling swift action against failing counterparties, ensuring operational efficiency and financial stability.
Stress testing and ensemble methods can fortify your credit portfolios. These techniques identify weaknesses under adverse scenarios and improve model accuracy through combined algorithm strengths. To comply with regulatory standards, align your models with ECB guidelines, and consider integrating XVA into your risk management framework. Combining these advanced approaches leads to robust and dynamic counterparty risk modeling, ideally suited to meet your company's precise needs.
To wrap things up, make sure your counterparty risk model covers financial health, credit history, industry analysis, operational risks, regulatory compliance, statistical risk estimation, and collateral security to achieve a robust evaluation.
First, you benefit from improved accuracy. Combining multiple models leverages the strengths of various algorithms, reducing errors and enhancing predictive power. This higher accuracy is crucial for robust counterparty risk assessments.
Second, you avoid overfitting. Techniques like bagging (e.g., Random Forests) reduce variance by training models on different data subsets in parallel. Boosting (e.g., AdaBoost), on the other hand, reduces bias by focusing on misclassified instances sequentially, giving you a more balanced model.
Third, you get increased robustness. Ensemble methods are more resilient to noisy and imbalanced data because they use averaging or voting mechanisms, making them less vulnerable to the weaknesses of individual models.
Fourth, you see bias and variance reduction. By combining different models, ensembles lower both bias and variance, resulting in more reliable and generalizable predictions. Methods like stacking and voting improve overall performance by addressing various training aspects.
Fifth, these methods handle complex data effectively. Ensembles adapt well to diverse counterparty risk scenarios, such as credit default prediction or creditworthiness assessment, enhancing the accuracy of your risk models.
Finally, in practical applications, you can apply ensemble methods to various risk models. These methods improve decision-making processes in financial institutions by providing more accurate and reliable risk assessments.
All things considered, using ensemble methods in your counterparty risk models delivers superior accuracy, robustness, and adaptability, empowering you to make better-informed financial decisions.
What Are The Key Components Of A Robust Counterparty Risk Model?
The key components of a robust counterparty risk model are essential for assessing creditworthiness and potential exposure. First, you need to analyze financial statements like balance sheets, income statements, and cash flow statements to gauge a counterparty’s financial health, profitability, and liquidity. Reviewing their credit history and payment behavior offers insight into their past performance and reliability. Assessing their industry dynamics, market position, and competitive landscape helps you understand the broader risks they face. Operational efficiency, risk management practices, and exposure to operational risks are also crucial. Evaluating their legal and regulatory compliance ensures they meet relevant laws and contractual obligations. Employing statistical tools to estimate the probability of default and potential loss prepares you for worst-case scenarios. Securing collateral and assigning a risk premium to transactions with higher default risk further mitigates potential losses. Incorporating both quantitative data and qualitative metrics ensures a comprehensive risk assessment.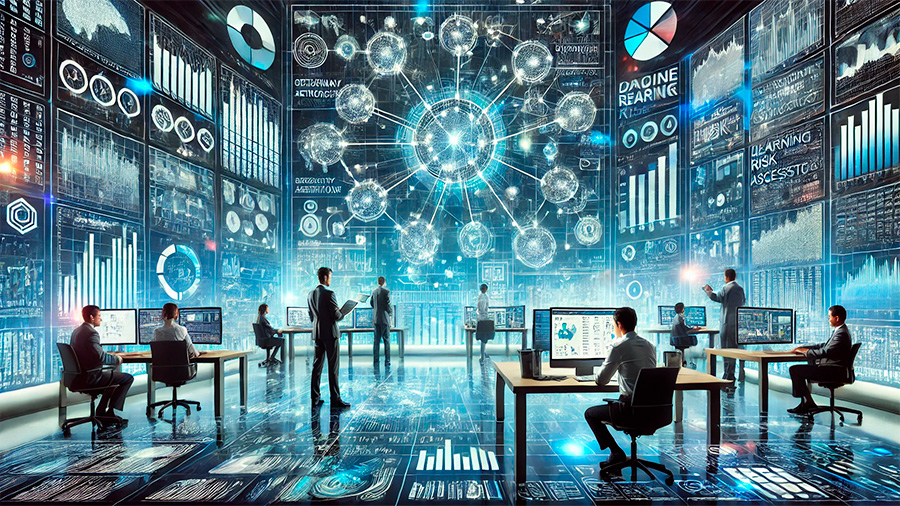
How Do Machine Learning Algorithms Enhance Counterparty Risk Assessment?
Machine learning (ML) algorithms enhance your counterparty risk assessment by improving accuracy, enabling real-time monitoring, and increasing efficiency. They analyze vast datasets, revealing hidden patterns and correlations that traditional methods might miss. This leads to more precise predictions of default probabilities and creditworthiness. You can benefit from ML algorithms that continuously monitor real-time data from various sources. This allows financial institutions to spot early warning signs and swiftly respond to changes in market conditions or customer behavior. By automating manual tasks, you streamline risk assessment, making the process faster and reducing human error.- Alternative Data and Behavioral Analytics: Incorporating alternative data sources and behavioral analytics provides a holistic view of a borrower's creditworthiness.
- Personalized Lending Decisions: Personalized lending decisions become more accurate and tailored.
- Enhanced Practices: Decision-making and risk management practices in the financial sector are significantly enhanced.
What Role Does Feature Engineering Play In Counterparty Risk Modeling?
Feature engineering plays a crucial role in counterparty risk modeling by creating, selecting, and transforming features from raw data to enhance model accuracy. You start by identifying relevant variables like financial history, macroeconomic indicators, or industry trends to predict a counterparty's risk of default.- Crafting Meaningful Features: Crafting meaningful features boosts the predictive power of your risk models, making them more accurate in forecasting defaults.
- Handling Missing Data: Handling missing data ensures your model remains robust and reliable despite incomplete information.
- Transforming Categorical Variables: Transforming categorical variables (like industry type) into numerical ones makes the data usable for machine learning algorithms.
- Enhanced Feature Sets: Enhanced feature sets lead to optimized decision-making on credit approvals, pricing, and portfolio management.
How Can Stress Testing Improve The Resilience Of Credit Portfolios?
Stress testing improves the resilience of your credit portfolios by helping you identify potential weaknesses and prepare for adverse economic conditions. By simulating severe scenarios, you gain insights into your risk exposure and can take proactive measures to mitigate potential losses.- Stress Testing: Stress testing reveals hidden vulnerabilities in your credit portfolio that might not be apparent during normal market conditions. You can see how specific events, like a housing market crash, might affect loan defaults and overall portfolio health.
- Evaluation of Risk Mitigation Strategies: It helps you evaluate the effectiveness of existing risk mitigation strategies. You might need to diversify your portfolio, adjust your risk appetite, or tighten underwriting standards based on the insights gained.
- Influence on Capital Requirements: Stress test results influence your capital requirements. If your portfolio shows potential losses, you may need to raise extra capital to comply with regulatory standards, ensuring you have a buffer to withstand financial shocks.
What Are The Benefits Of Using Ensemble Methods In Counterparty Risk Models?
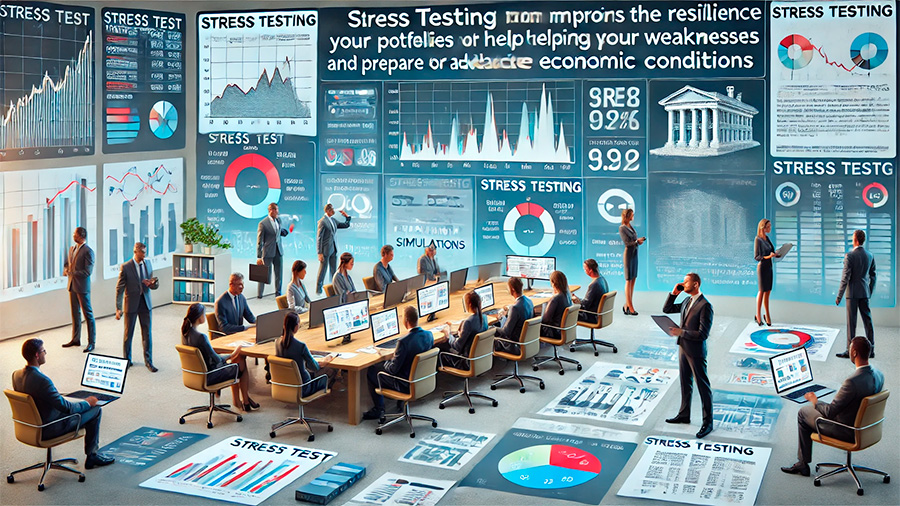